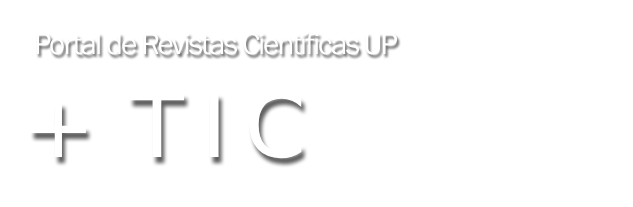
Artículos
In recent years, the growth of Machine Learning has had a significant impact across various fields, including education. In the educational sphere, Machine Learning techniques or algorithms have been applied for various purposes, such as predicting the academic performance of higher education students. This article focuses on reviewing publications that present the results obtained by employing Machine Learning algorithms to predict the academic performance of university students. A systematic review was conducted using academic databases such as Redalyc, Google Scholar, and Scielo, utilizing search filters and relevant keywords. Subsequently, the most relevant articles were selected for analysis. The purpose of this review article is to identify the most frequently used and effective Machine Learning algorithms for predicting the academic performance of university students, as well as to examine how they have been implemented in different universities, serving as a guide for future research involving the application of these algorithms with student data from the University of Panama. The results show that the most commonly used algorithms for this type of prediction are Decision Trees, K-Nearest Neighbors (KNN), Naive Bayes, Random Forest, and Support Vector Machines (SVM), as well as Artificial Neural Networks, which also stand out for their high accuracy. The use of these algorithms to predict academic performance in universities offers great potential to reduce dropout rates, improve academic outcomes, support pedagogical decisions, and optimize educational resources.